If you’ve ever worked at an organization of human beings (or particularly smart chimps), you’ve likely noticed that the way decisions are made is mysterious. Indeed, “made” is too strong a word. Decisions emerge from a complex stew of strategic, political, and personal incentives that AI will struggle to replicate for centuries to come, if only because AI could never be so irrational.
But mysterious isn’t the same as random. After many years of observation and experience, I’ve noticed a pattern in how smooth decision-making tends to be, and it’s a function of data: Namely, the amount of data readily available to inform a decision.
So here it is — a theory that will no doubt echo in the halls of history like Einstein’s general theory of relativity…
The horseshoe theory of organizational decision-making
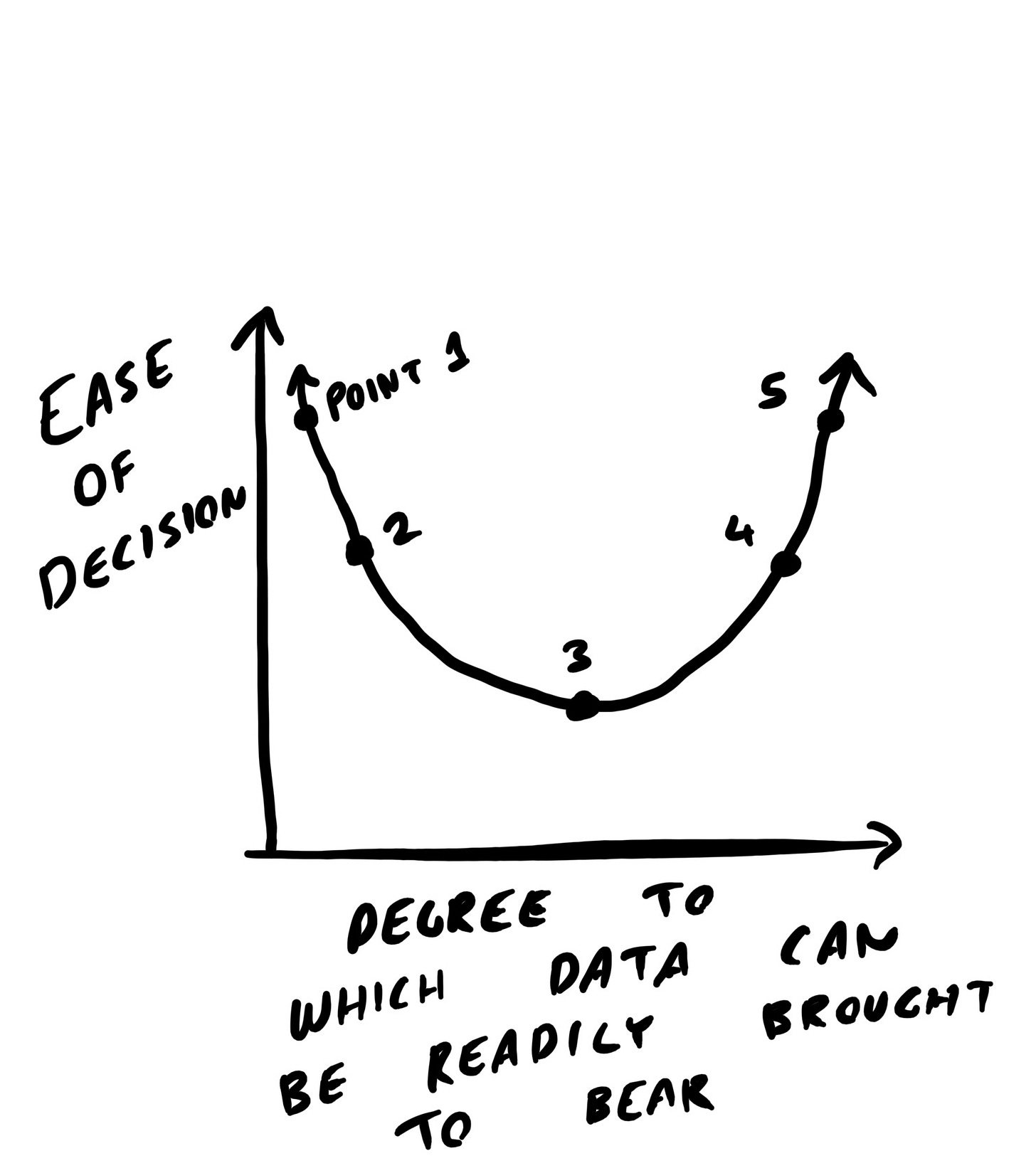
Let's delve deeper into the theory's implications.
Points 1 and 2: Assign a decision-maker and carry on
Points 1 and 2 represent decisions where little to no relevant data is available. In these instances, it should be clear to all involved that decisions must be made by a specific person — probably a HiPPO — using qualitative rather than quantitative judgment. These decisions can be big or small, from launching a blue-sky initiative to deciding on the color of a new page’s call-to-action. (Of course, if you’re Google, it could be worth A/B testing the latter; the rest of us will use the style guide and get on with life.)
HiPPOs are funny creatures. They seem cute but are, in fact, quite dangerous and mercurial — whoop, sorry! ChatGPT got confused trying to complete this paragraph. It thought I was talking about actual hippos, not an organization’s Highest Paid Persons, who are comparably dangerous and mercurial, but less cute.
Some human HiPPOs are okay — even happy — to take charge of decision-making. (These are the ones who devoured Steve Jobs’s biography in business school.) But others are self-conscious or even scared of their power. Beware of these HiPPOs, particularly if you’re a data scientist. They may attempt to turn a qualitative decision into a faux-data-driven one. The resulting quagmire always saps morale.
Points 4 and 5: Analyze the data you need and carry on
Conversely, Points 4 and 5 represent decisions that meet two criteria:
They are unambiguously data-driven.
Data can be readily brought to bear on the decision. (This second criterion is crucial: Stakeholders often underestimate the effort required to make data accessible; part of the data team's job is to remind them.)
The initiatives in this part of the graph include A/B tests and anything that’s particularly amenable to a well-scoped analysis question (e.g., “What’s the leakiest part of our funnel?” or “Should we expand the marketing campaign we launched in Canada to the US?”)
These problems are the bread-and-butter of any data scientist’s career. And whether you’re dealing with sourdough, wheat, or rye, butter is always a delicious addition — whoop, sorry! ChatGPT got confused again!
What ChatGPT meant to say was that the standard failure modes of Points 4 and 5 are often cultural rather than technical. On the one hand, some organizations may shy away from relying on data because they don’t like it when reality messes with their perceptions:
On the other hand, some organizations become excessively data-driven, sapping their workforce of ambition and vitality, ultimately leading them to local rather than global optima:
Neither is good!
As challenging as it can sometimes be to make decisions on Points 1, 2, 4, and 5, I've discovered the most existential peril lurks on Point 3...
The trough of the decision-making horseshoe
"In The Trough" (also the title of my upcoming data-themed horror film), there's usually just enough data floating around to make even the most Jobsian HiPPOs hesitant about making swift decisions: What if another HiPPO brings up this information at the watering hole/executive sync? Even if the data is a complete red herring, they don't want to look silly!
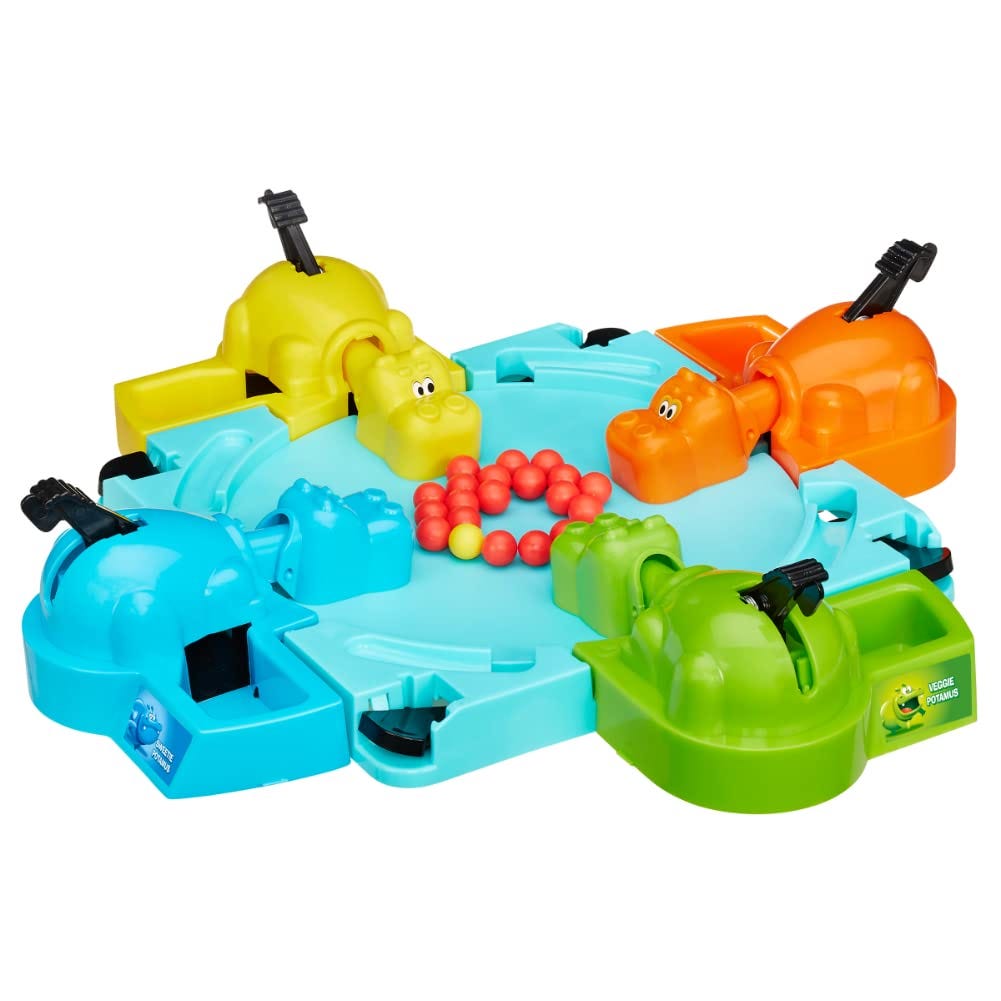
Meanwhile, many data scientists also feel anxious about trough-y decisions. They know that their teams look to them to make the unknowable more knowable. At the same time, they worry that an overreliance on data might slow things down or constrain their team’s search space in counterproductive ways.
What is our data scientist to do?
Well, in my screenplay for "In The Trough," they need to collaborate with the Ghost of Tech-Debt Past to repair a haunted data pipeline wreaking havoc on their team's Jupyter Notebooks.
But in reality, they should proactively work to reshape the horseshoe. In the words of essential Twitter follow Katie Bauer, that doesn’t imply a narrow focus on decision-making, per se. It means thinking holistically about making data valuable:


For instance, by prioritizing the creation of clean, well-documented, and well-maintained data sets, along with the unglamorous but vital work of dashboarding and automating common analyses, decisions that used to occupy Points 3 can be shifted to Points 4 and 5. Integrating data into an organization's decision-making loop is as much about convenience and velocity as it is about rigor.
Data scientists can also expand their methodological toolkits. They can learn causal inference (shameless plug: I’m teaching a causal inference course on CoRise!). They can engage with their qualitative research colleagues (my favorite people!). When the data team’s capabilities and relationships deepen, so does their ability to guide more complex decisions.
Most importantly, data scientists should internalize the fact that their job isn't merely to “do data science." It's about educating and training their organizations to become better decision-making machines. That means being the person who consistently considers the power and limitations of data — when it's useful and when it might constrain or even mislead.
This may seem like an obvious point, but I've found that data scientists often struggle to ask the critical follow-up questions that prevent decision-making quagmires from forming in the first place, e.g., "That's an interesting question, Stakeholder! How would the answer inform your ultimate decision?" or "Here's my recommendation, but given the data we have, I'm only 75% sure and the error bars are wide. Do we need more precision?" or "We might be able to answer this, but it'll be rough and take a week. Is the decision important enough to wait for the analysis, or should we make a call now?"
Some of these questions may seem counterproductive, but I've found they benefit both the team and the individual. The team knows they have a reliable data partner, and the individual — perhaps unexpectedly — discovers they're more empowered to influence their team's strategic direction. Relationships become two-way, not one-way. That enables a far more proactive orientation on the part of data.
Of course, none of this is groundbreaking. Nevertheless, I hope the horseshoe provides a helpful theoretical grounding for thinking through decision-making and the role of data therein.
After all, even if quagmires are occasionally unavoidable, a decision-making V is still better than a decision-making U!
So, the next time you find yourself in a decision-making meeting going haywire, pause and ask yourself: Are we in the trough, and can we climb out?
Also, please call Netflix and ask them to greenlight my movie.